Social media posts can predict diabetes, depression, anxiety, and psychosis better than demographic info: Study
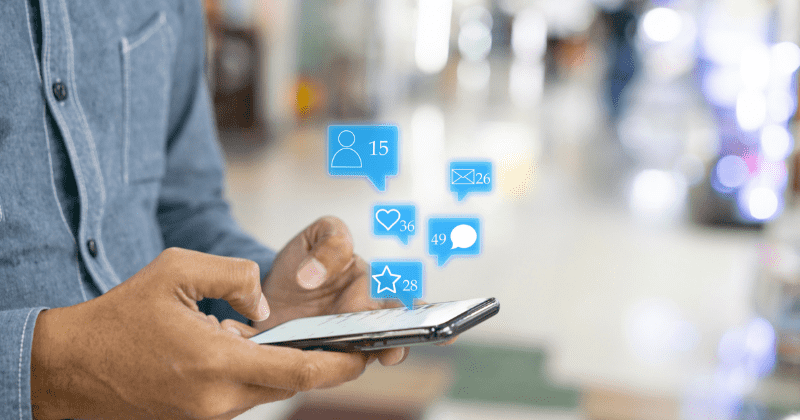
Facebook posts can be used to predict diabetes and mental health conditions such as depression, anxiety, and psychosis, according to a new study.
The paper, published in PLOS One, analyzed 949,530 Facebook status updates containing 20,248,122 words across 999 consenting participants, whose posts included at least 500 words.
The research team analyzed whether medical conditions across 21 broad categories were predictable from social media content.
They found all 21 medical conditions could be predicted from the language used in the Facebook posts.
Analysis reveals that language used in Facebook posts are particularly effective at predicting diabetes and mental health conditions, including anxiety, depression and psychoses, when compared to demographic information.
Significantly, the findings show that 10 medical conditions were better predicted by Facebook language alone as compared to standard demographic factors such as age, sex, and race.
“Over two billion people regularly share information about their daily lives over social media, often revealing who they are, including their sentiments, personality, demographics, and population behavior. Because such content is constantly being created outside the context of health care systems and clinical studies, it can reveal disease markers in patients’ daily lives that are otherwise invisible to clinicians and medical researchers. In what we believe to be the first report linking electronic medical record data with social media data from consenting patients, we identified that patients’ Facebook status updates could predict many health conditions, suggesting opportunities to use social media data to determine disease onset or exacerbation and to conduct social media-based health interventions,” the researchers said in the paper.
The team comprised researchers from Penn Medicine Center for Digital Health, University of Pennsylvania; Department of Computer Science, Stony Brook University; Department of Emergency Medicine, Perelman School of Medicine, University of Pennsylvania; The Center for Health Equity Research and Promotion-Philadelphia Veterans Affairs Medical Center; The Wharton School, University of Pennsylvania; Positive Psychology Center, University of Pennsylvania; Department of Computer and Information Science, University of Pennsylvania, and Microsoft Research, New York.
The researchers linked electronic medical records (EMRs) of consenting patients with their social media data to ascertain if they could predict individuals’ medical diagnoses from language posted on social media and if they could identify specific disease markers from social media posts.
Using an automated data collection technique, the researchers analyzed the Facebook post history of consenting patients.
The team then built three models to analyze their predictive power for the patients: one model assessed the Facebook post language, another used demographics such as age and sex, and the last combined the two datasets.
“This is the first study to show that language on Facebook can predict diagnoses within people’s health record, revealing new opportunities to personalize care and understand how patients’ ordinary daily lives relate to their health,” the researchers say.
“The medical condition categories for which Facebook statuses show the largest prediction accuracy gains over demographics include diabetes, pregnancy, and the mental health categories anxiety, psychoses, and depression,” states the paper.
The study covered 21 medical conditions:
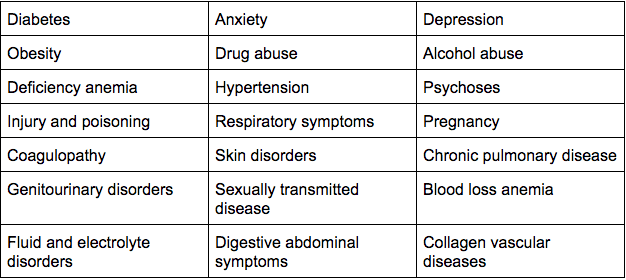
The researchers say that several topic markers of diagnoses reveal specific behavior or symptoms.
For example, alcohol abuse was marked by a topic mentioning drink, drunk, and bottle. Issues or words that expressed hostility such as dumb and certain expletives served as indicators of drug abuse and psychoses. “Topics most associated with depression suggested somatization (for example, stomach, head, hurt) and emotional distress (for example, pain, crying, tears),” states the paper.
There were other interesting findings; for example, diabetes was predicted by religious language (for example, god, family, pray). “This does not mean that everyone mentioning these topics has the condition, but just that those mentioning it are more likely to have it. For example, the top 25% of patients mentioning the (god, family, pray) topic were 15 times more likely to have been diagnosed with diabetes than those in the bottom 25% of mentioning that same topic. This association may be specific to our patient cohort and suggests the potential to explore the role of religion in diabetes management or control,” the researchers say.
The researchers say while social media has a lot of potential to personalize healthcare as people’s personalities, their mental state, and health behaviors are reflected in their social media, the latter’s power to predict diagnosis also raises questions regarding privacy, data ownership, and informed consent.
The research team adds that efforts should be made to ensure that users know how their data can be used and how they can recall such data.