Artificial intelligence could be just as accurate in detecting diseases as health professionals, says study
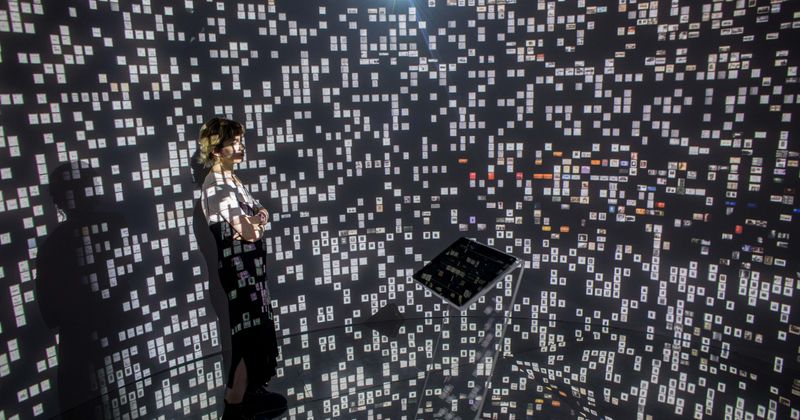
Artificial intelligence (AI) could be as effective as health professionals at diagnosing diseases, according to a review of evidence from scientific literature.
Analyzing data from 14 studies, a research team compared the performance of deep learning — a subset of AI — with humans in the same sample. They found that deep learning algorithms can correctly detect diseases in 87% of cases, as compared to 86% achieved by healthcare professionals.
Deep learning utilizes algorithms, big data, and computing power to emulate human learning and intelligence, stated the study published in The Lancet Digital Health journal.
"From this exploratory meta-analysis, we cautiously state that the accuracy of deep learning algorithms is equivalent to healthcare professionals, while acknowledging that more studies considering the integration of such algorithms in real-world settings are needed," says the study.
The ability to accurately exclude patients who do not have disease was also similar for deep learning algorithms (93%) as compared to healthcare professionals (91%), says the research team led by Professor Alastair Denniston from University Hospitals Birmingham NHS Foundation Trust, UK.
"Within a handful of high-quality studies, we found that deep learning could indeed detect diseases ranging from cancers to eye diseases as accurately as health professionals. But it is important to note that AI did not substantially outperform human diagnosis," says Professor Denniston.
The study
Deep learning is a form of artificial intelligence that offers considerable promise for improving the accuracy and speed of diagnosis through medical imaging. With deep learning, computers can examine thousands of medical images to identify patterns of disease.
"Medical imaging is one of the most valuable sources of diagnostic information but is dependent on human interpretation and subject to increasing resource challenges," says the research team.
"The need for, and availability of, diagnostic images is rapidly exceeding the capacity of available specialists, particularly in low-income and middle-income countries," the team adds.
"Automated diagnosis from medical imaging through AI, especially in the sub-field of deep learning, might be able to address this problem," the study states.
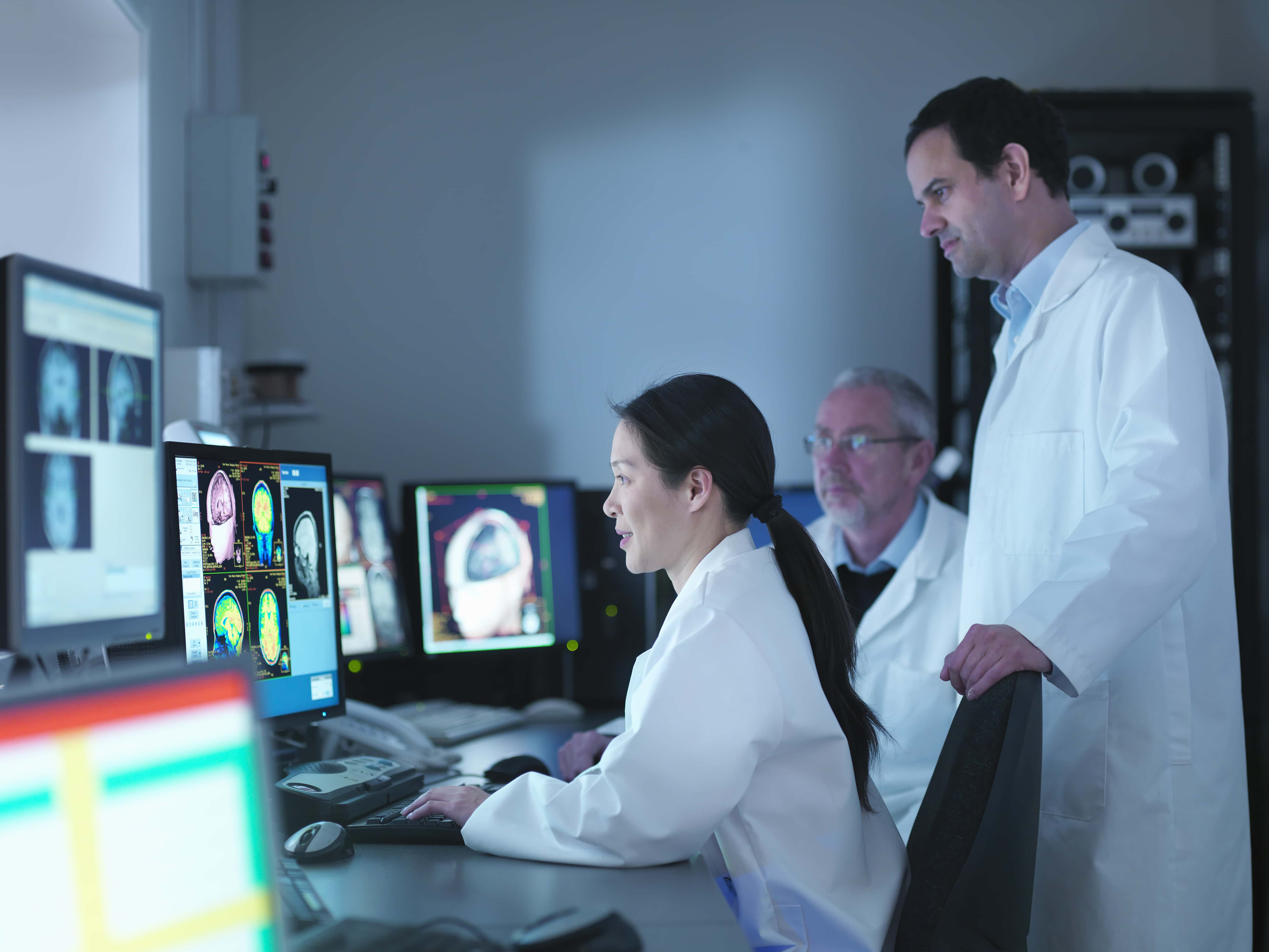
There is a substantial public interest and market forces that are driving the rapid development of such diagnostic technologies. Reports of deep learning models outperforming humans in diagnostic testing have generated much excitement and debate.
More than 30 AI algorithms for healthcare have already been approved by the US Food and Drug Administration (FDA). However, concerns have been raised about whether study designs are biased in favor of machine learning, and the degree to which the findings are applicable to real-world clinical practice.
To provide more evidence, the team conducted a systematic review and meta-analysis of all studies comparing the performance of deep learning models and health professionals in detecting diseases from medical imaging published between January 2012 and June 2019. They also evaluated study design, reporting, and clinical value.
"There is an inherent tension between the desire to use new, potentially life-saving diagnostics and the imperative to develop high-quality evidence in a way that can benefit patients and health systems in clinical practice," says Dr. Xiaoxuan Liu from the University of Birmingham, UK.
"A key lesson from our work is that in AI, as with any other part of healthcare, good study design matters. Without it, you can easily introduce bias which skews your results," Dr. Liu points out.
"These biases can lead to exaggerated claims of good performance for AI tools which do not translate into the real world. Good design and reporting of these studies is a key part of ensuring that the AI interventions that come through to patients are safe and effective," Dr. Liu adds.
The analysis
Researchers identified 31,587 records of which 20,530 were screened. In all, 82 articles met the eligibility criteria for the systematic review, and only 25 met their inclusion criteria for the meta-analysis.
These 25 studies compared the performance of deep learning solutions to healthcare professionals for 13 different specialty areas, only two of which — breast cancer and dermatological cancers — were represented by more than three studies.
"We reviewed over 20,500 articles, but less than 1% of these were sufficiently robust in their design and reporting that independent reviewers had high confidence in their claims," says Professor Denniston.
"What's more, only 25 studies validated the AI models externally (using medical images from a different population), and just 14 studies actually compared the performance of AI and health professionals using the same test sample," the professor adds.
The diagnostic performance of deep learning models in the review of 14 studies matched that of healthcare professionals. "Deep learning offers considerable promise for medical diagnostics," says the team.
"We aimed to evaluate the diagnostic accuracy of deep learning algorithms versus healthcare professionals in classifying diseases using medical imaging. Our review found the diagnostic performance of deep learning models to be equivalent to that of healthcare professionals," the team adds.
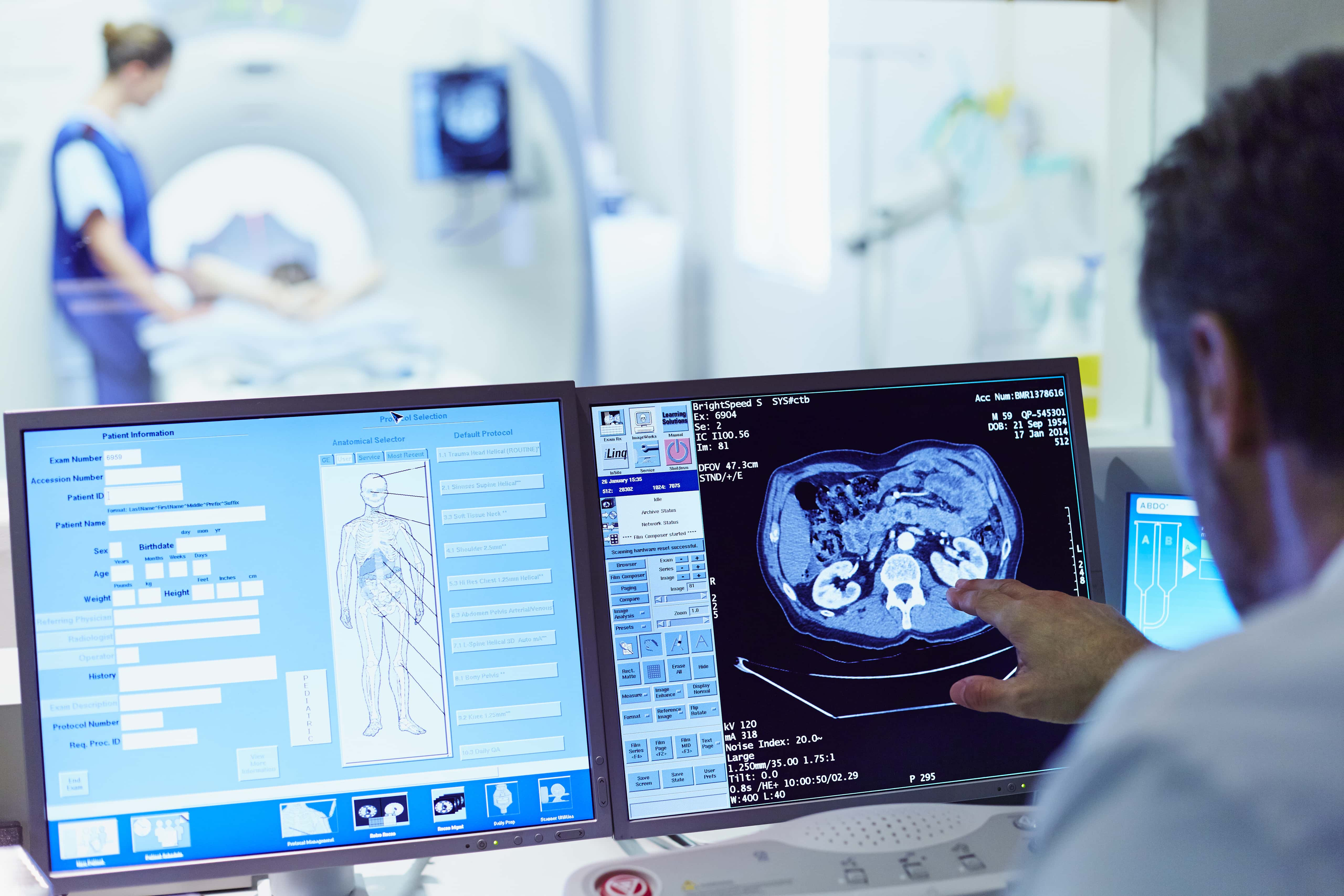
However, since only a few studies were of sufficient quality to be included in the analysis, researchers caution that the true diagnostic power of the AI technique, deep learning, remains uncertain.
They explain that this is because of the lack of studies that directly compare the performance of humans and machines, or that validate AI's performance in real clinical environments.
The limitations
According to the research team, there are many limitations in the studies they analyzed. Deep learning was frequently assessed in isolation in a way that does not reflect clinical practice.
"For example, only four studies provided health professionals with additional clinical information that they would normally use to make a diagnosis in clinical practice," says the study.
The researchers say that evidence on how AI algorithms will change patient outcomes needs to come from comparisons with alternative diagnostic tests in randomized controlled trials.
"So far, there are hardly any such trials where diagnostic decisions made by an AI algorithm are acted upon to see what then happens to outcomes which really matter to patients, like timely treatment, time to discharge from hospital, or even survival rates," says Dr. Livia Faes from Moorfields Eye Hospital, London.
Writing in a linked commentary, Dr. Tessa Cook from the University of Pennsylvania, USA, says that with the increasing hype of the potential of AI in medicine, the results of the current study — which concludes that the accuracy of deep learning is similar to that of healthcare professionals — could be misconstrued as machine diagnosis being better than human diagnosis.
"Why have a human doctor when a digital one would be just as good, maybe better? Given the extensive discussion of the limitations of their study, claiming equivalence or superiority of AI over humans could be premature," says Dr. Cook.
"Perhaps the better conclusion is that, in the narrow public body of work comparing AI with human physicians, AI is no worse than humans, but the data are sparse, and it might be too soon to tell," Dr. Cook adds.